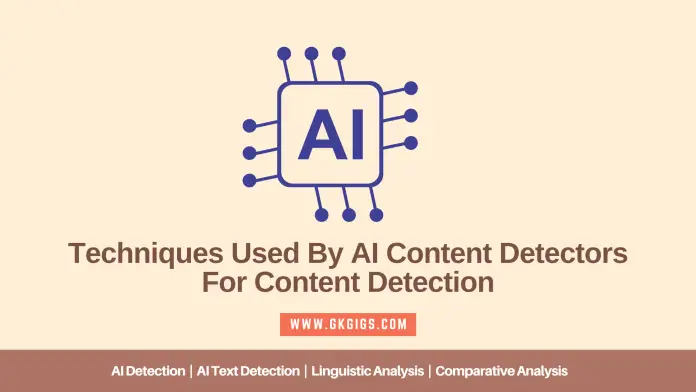
Last Updated: 23 August 2023
Today, the line between human-generated content and machine-generated content is growing increasingly blurry. Artificial Intelligence (AI) systems can now produce articles, stories, and reviews that are often indistinguishable from those penned by human authors.
While AI has proven to be a boon for automating tasks and generating new content, it also raises questions about authenticity and credibility. How can we tell if the text we are reading was crafted by a human being or whipped up by a computer program?
This is where an AI content detector comes to our rescue. These smart tools are designed to discern human-created content from machine-generated pieces, ensuring the integrity and reliability of information.
In this article, we will unveil five useful techniques that an AI content detector employs to perform this essential task.
So, buckle up as we take a deep dive into the intelligent world of AI content detection!
Page Contents
What is AI Detection?
AI Detection refers to the process of distinguishing between text written by a human and text generated by a computer program, or Artificial Intelligence. At the heart of this process are classifiers, which are essentially tools or algorithms trained to sort data into various categories. In the context of AI Detection, these classifiers are adept at segregating human-written content from AI-generated material.
To achieve this, classifiers utilize machine learning algorithms. These algorithms analyze the different features of a piece of text, such as its syntax, semantics, and style.
Based on this analysis, the classifier assigns a confidence score to the text—a numerical value that indicates how likely it is that an AI wrote the content. A high confidence score suggests that the text is probably machine-generated, while a low score indicates that a human likely wrote it.
Why is AI Text Detection Important?
AI text detection serves as a sentinel, guarding the credibility of the information we consume daily. This is especially significant in fields like SEO, academia, and law. For instance:
- In SEO, the authenticity of content affects website rankings—a critical factor for businesses striving for visibility.
- In academia, where the merit of work is heavily reliant on original thought and research, AI text detection helps in upholding the integrity of scholarly publications.
- In legal contexts, ensuring that advice and documents are human-generated and carefully crafted is essential to avoid misinformation and potential harm.
The consequences of undetected AI-generated content can be dire. For example, consider ‘Your Money or Your Life’ (YMYL) topics—those that can impact a reader’s health, finances, or safety. If unchecked AI starts writing about medical treatments or financial advice, the misinformation could lead to serious harm.
How AI Detection Works for AI Content Detection?
Determining the origin of the content—whether human or machine—is quite a task. But certain techniques have been developed to make this process precise and efficient. Here’s a deep dive into how an AI content detector tool like the one offered by Paraphrasingtool.ai functions:
1. Linguistic Analysis
The heart and soul of any text is its language. Through linguistic analysis, AI detectors can examine various components of a text to ascertain its origin.
- Examining sentence structure: Just as we all have unique ways of constructing our sentences, AI-generated content has certain tells. The way a sentence is structured, the order of its clauses, and even its length can be indicators.
- Detection of semantic meaning: While humans tend to maintain a consistent thematic flow, AI might sometimes stray, inserting sentences that are syntactically correct but semantically off.
- Spotting repetitions or anomalies in text: AI often leans on certain phrases or structures it has been trained on, leading to noticeable repetitions.
2. Comparative Analysis
Here’s where history becomes handy. By comparing a piece of text with known samples, we can often determine its provenance.
- Comparison with known AI-generated and human-written texts (training datasets): By maintaining vast databases of known texts, AI detectors can find matches or similarities to indicate a text’s source.
- Identification of similarities and differences: This isn’t just about matching words but also styles, tone, and even the order of information presentation.
3. Specific Techniques Used by AI Content Detectors
Beyond these broad categories, there are specific techniques that are instrumental in this detection process.
I. Classifiers for AI Detection
A classifier acts as a sorting machine in the realm of machine learning, categorizing data into predefined classes based on certain features—much like sorting apples by their color. In the context of AI detection, classifiers are trained to distinguish between human-written and AI-generated text using features such as the words used, grammar, style, and tone of the writing.
- Supervised Classifiers: These are trained using labeled data—datasets where each example is tagged as either AI-generated or human-written. This gives the algorithm a reference point for what each category looks like.
- Unsupervised Classifiers: Unlike their supervised counterparts, these classifiers are unleashed on unlabeled data. They must figure out the patterns and groupings in the data independently.
For example, imagine a scenario where a classifier is trained to differentiate between human-written book reviews and those generated by a computer program. The classifier would learn the nuanced expressions and sentiments generally found in human reviews and use this knowledge to categorize new, unseen reviews.
II. Using Embeddings for Detection
In the world of AI and Natural Language Processing (NLP), embeddings are like digital fingerprints for words or phrases. They are high-dimensional vectors that capture the essence and relational context of words, and they can be incredibly useful for detecting AI-generated content.
- Word Frequency Used for Detection: Word frequency analysis involves studying how often certain words appear in a given text. For instance, if “AI technology” is repeatedly and unnaturally used in an article, it might signal that the content was generated by an AI model.
- N-gram Analysis Used for Detection: N-grams refer to contiguous sequences of ‘N’ items from a given text. With N-gram analysis, the system detects patterns in such sequences and uses these patterns to help identify the origin of the text. For instance, AI-generated text might use certain three-word sequences (trigrams) more frequently than human writers do.
- Syntactic Analysis Used for Detection: Syntactic analysis dives into the grammatical structure of sentences, looking at the arrangement of words and phrases. For example, while “The dog chased the cat” and “Chased the dog, the cat” use the same words, their meanings differ drastically due to syntax.
- Semantic Analysis for Detection: The semantic analysis goes beyond the structure of the text and delves into its meaning. It aims to understand the themes, topics, and sentiments of the content. Inconsistent or illogical flows of ideas can be a sign that the text was machine-generated.
III. Perplexity Used for Detection
Perplexity is like a measure of a text’s predictability. For AI detection, it evaluates how similar a piece of text is to the data the AI was trained on. A lower perplexity suggests that the text closely resembles the AI’s training data, indicating that it is likely AI-generated. On the contrary, more complex, unpredictable text—typical of human writers—will result in higher perplexity.
For example, compare a nuanced, intricate human-written statement on climate change with a more predictable, straightforward AI-generated statement. The human-written text would likely have a higher perplexity value.
IV. Detection by Burstiness
‘Burstiness’ refers to the sudden, repeated appearance of specific words or phrases in a text. AI models, when generating text, may overuse certain phrases they were heavily exposed to during their training. Detecting such ‘bursts’ of repeated terms can be a strong indicator that the text was machine-generated.
For example, if an article about space exploration inexplicably keeps using the phrase “quantum physics” in almost every paragraph without a clear context, that could be a burstiness red flag—a sign that the content might have been generated by an AI model.
To Sum Up
So, I’ve covered useful techniques AI content detectors use for content detection. We’ve delved into why you might need such a tool, what really goes on behind the scenes when training such a model, and how to interpret its accuracy.
I hope this deep dive helps you understand this topic slightly better and that it proves useful for you.
Read More |
- Computer General Knowledge (Basic To Advanced)
- List Of Top Computer Companies And Their Founders
- Common Problems With Binary Translation and Solutions
- List Of All Social Networking Sites And Their Founders (2023)